Nvidia Exec: AI Rides on Symbiotic Hardware-Software Relationship
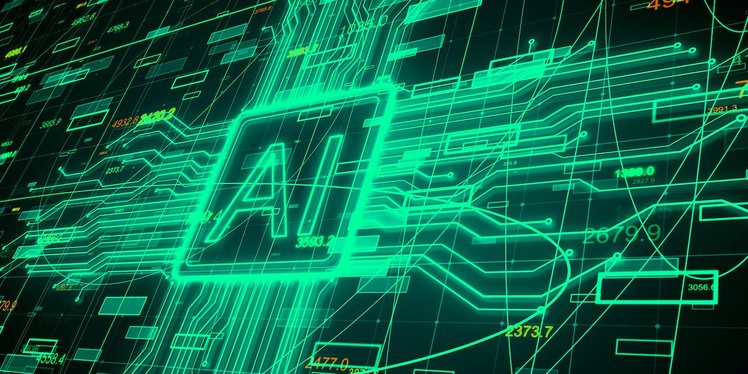
Software dominated Nvidia’s fall GTC conference and for good reason, Manuvir Das, head of enterprise computing at Nvidia, told SDxCentral. While hardware like Nvidia’s GPUs and artificial intelligence (AI) accelerators are essential to enabling AI workloads, it’s only half the equation, he said.
The other half is, of course, the software libraries and frameworks that actually make the hardware useful. The chipmaker has invested heavily in recent years to expand its software ecosystem to this end.
“Nvidia doesn’t just make hardware or software, it’s a full-stack company,” Das said. “We build all the layers of software and all the layers of hardware.”
AI is Do or Die
These software frameworks are critical, according to Das, as AI becomes a do-or-die phenomenon for enterprises. “Regardless of the industry you’re in, if you don’t adopt AI now … you will be left behind,” he said. “You need to embrace AI because your competitors who have embraced AI are already leveraging it to build better products.” While the imperative for AI is clear, adoption remains low, Das said.
Dell’Oro Group analyst Baron Fung estimates fewer than 2% of the servers shipped to enterprises on a unit basis are equipped with an AI accelerator like a GPU.
This is due in part to the ability to run AI workloads in the cloud, he explained. “The cloud service providers can drive better utilization and efficiencies than the enterprise ever can. … This is the premise of the cloud computing model, and it’s applicable to AI as well.”
While AI has seen wider adoption in the cloud, where enterprises can forgo the extreme upfront cost of AI hardware, Das argues many large enterprises are hesitant to deploy their workloads on infrastructure they don’t own or control.
“What we see more and more, as companies are adopting AI, is that they’re very interested in doing AI in their own data centers,” he said.
Eliminating AI Barriers
On premises AI adoption has been slowed by a number of factors including access to quality data, data scientists, AI infrastructure, and the software necessary to productize that data, according to Das.
“If you don’t have the right data, then there’s nothing you can do,” he said, adding that even after enterprises have solved the data collection and storage problem, they’re still left with a daunting infrastructure challenge.
Data center infrastructure is expensive, but the highly specialized hardware used in supercomputing clusters such as Nvidia’s Selene are an order of magnitude more expensive. A single Nvidia DGX A100 server will set an enterprise back as much as $199,000 and, depending on the size of the AI model, multiple racks of these systems may be required.
“Each GPU server can be ten times the cost — or more — than that of a general purpose server,” Fung told SDxCentral. And that doesn’t take into account the specialized infrastructure required to support it. “Unless the enterprise can scale and fully utilize these AI deployments, the economics for on-premise deployments may be harder to justify.”
This poses a real challenge for enterprise IT teams, which may need to deploy AI capabilities within their existing data center footprint using traditional networking and storage architectures, Das explained.
“In this environment, you’re talking about systems and tools that are very different from the world Nvidia came from,” he said. “They’re using all these standard servers that cost $8,000 whereas the kinds of systems we build with DGX are much more expensive.”
This is where Nvidia’s EGX platform — which pairs industry-standard server chassis from vendors like Dell, Hewlett Packard Enterprise, or Lenovo with the chipmaker’s GPUs — comes in.
These servers are designed to be deployed in brownfield data centers using standard Ethernet networks.
Justifying the Cost
Once enterprises address the infrastructure challenge, enterprises have to identify low-hanging fruit that will deliver the fastest return on their investment, according to Das.
This is where Nvidia has spent the lion’s share of its time in recent years building out software frameworks that package common data analytics and machine learning libraries into a ready-to-consume package.
“These software frameworks contain all the plumbing and things you need underneath so that you can just adopt the framework,” Das said. “There’s a little bit of wrapper code you put around them, and they’re ready to go for a use case.”
A company that wants to implement natural-language processing for a customer service chat bot, for example, might use Nvidia’s RIVA framework, he explained. There are dozens of frameworks that address everything from same-day delivery routing to zero-trust computing architectures, he added.
Essentially, anywhere there’s an opportunity to accelerate a data-intensive task, Nvidia is developing a software framework, according to Das.
And following the debut of Nvidia AI Launchpad, customers can start developing AI applications using these products before they’ve acquired the hardware to run them.
AI Launchpad provides pre-provisioned hardware and software in nine locations around the globe. “The idea is just come in, use it, and experience it, and see what it can do for you,” Das said.
What Does the Future Hold for the AI Enterprise?
In the coming years, Das expects AI to become commonplace in the enterprise.
“Almost every enterprise company today has a team of people who are virtualization experts,” he said. “The shift we’re looking forward to for enterprise companies is when AI is thought of the same way.”
AI workloads mostly run in large, centralized supercomputing clusters today, but Das expects these workloads to eventually make their way out to the edge of the network.
“If you look any number of years out at the enterprise data center, much of it will be what we think of as the edge,” he said. “A few servers in a closet somewhere, rather than a big building with thousands of servers.”
These distributed AI networks will also enable a new wave of low-latency workloads because they can be located in close proximity to the user rather than backhauling data to the data center, Das explained.